How Data Science is Revolutionizing the Education Industry
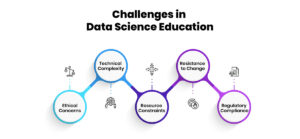
Introduction
In today’s digital world, data is everywhere—and education is no exception. From online learning platforms to classroom tools, data science is changing the way we teach and learn. By analyzing huge amounts of educational data, schools and edtech companies are personalizing learning, improving student outcomes, and making smarter decisions.
Gone are the days of one-size-fits-all education. Thanks to data science, learning is now more customized, effective, and accessible than ever before. It’s not just about crunching numbers—it’s about using those insights to help students succeed.
Data-Driven Personalization in Learning
Custom Learning Paths for Every Student
Just like Netflix recommends shows based on your viewing history, modern learning platforms can suggest lessons based on a student’s progress, strengths, and areas of struggle.
Example: Duolingo
The popular language-learning app uses data science to track how quickly you learn, where you struggle, and when you’re likely to forget something. Then, it adjusts the lessons to help you stay on track.
How It Works:
- Machine Learning Algorithms: Track user activity and performance.
- Predictive Models: Anticipate when a student might need a review.
- Adaptive Learning Engines: Serve up personalized content in real-time.
Why It Matters:
This approach keeps students engaged, gives them the support they need, and increases the likelihood of achieving their learning goals.
Predicting Student Performance and Preventing Dropouts
Early Intervention with Predictive Analytics
Teachers no longer have to wait until the end of a semester to see who’s falling behind. Data science allows educators to spot at-risk students early, giving them a chance to step in before problems escalate.
Case Study: Georgia State University
Georgia State University uses predictive analytics to monitor over 800 factors for each student, like grades, attendance, and class engagement. When a student shows signs of struggling, advisors are alerted, allowing them to offer support—leading to dramatically higher graduation rates.
Techniques Involved:
- Time-Series Analysis: Tracks student behavior over time.
- Classification Models: Predicts which students may struggle based on past patterns.
- Natural Language Processing (NLP): Analyzes written feedback, essays, or discussion posts for emotional and academic signals.
Smart Content Creation and Curriculum Design
What Should We Teach Next?
Curriculum developers can now use data science to decide what content works and what doesn’t, based on student engagement and performance.
Example: Khan Academy
Khan Academy analyzes how long students spend on videos, where they pause or rewatch, and which problems they struggle with. This data helps the platform refine its lessons to better fit students’ learning patterns.
AI Tools for Teachers:
- Content Heatmaps: Show which lessons are most engaging.
- Feedback Analytics: Identify which topics need more clarification.
- AI-Generated Question Tools: Auto-create quizzes based on the content.
Result:
This leads to better content, higher engagement, and improved learning outcomes.
Improving Teacher Effectiveness
Data-Backed Teaching Insights
Data isn’t just helpful for students—it’s also a valuable tool for teachers. With dashboards that provide real-time insights into class performance, educators can quickly adjust their approach to meet the needs of their students.
Example: Google Classroom Analytics Plugins
Teachers can use Google Classroom’s analytics tools to track class trends, monitor student participation, and identify which students might need extra help—all without having to dig through piles of graded assignments.
Enhancing EdTech Products with Data Science
Smarter Platforms, Happier Users
Data science is also the backbone of successful edtech products. Whether it’s optimizing user experience or improving conversion rates, data helps make these platforms more effective.
Example: Coursera
Coursera uses A/B testing and behavior analytics to improve its course layouts, recommendation engines, and mobile experience, ensuring that students have a smooth and effective learning journey.
Techniques Used:
- Behavioral Clustering: Groups similar learners for targeted content.
- Churn Prediction: Helps retain users by predicting who might drop off.
- Reinforcement Learning: Refines content suggestions based on user feedback.
Data Science for Educational Equity
Closing the Gap One of the most powerful uses of data in education is promoting fairness. Data science helps identify achievement gaps based on factors like region, socioeconomic status, or race. This allows schools and governments to take action to ensure all students have equal access to quality education.
Example: UNESCO & AI for Education
UNESCO partners with AI researchers to analyze global education data and create policies aimed at improving access to education for underserved communities.
The Future of Data Science in Education
As technology continues to evolve, data science will play an even bigger role in education. Here’s what we can expect:
- Hyper-Personalized Learning: Education tailored to every student’s pace, style, and goals.
- Real-Time Dashboards: For students, teachers, and parents to monitor progress.
- Emotion-Aware AI: Lessons that adjust based on a student’s mood or engagement level.
- Lifelong Learning Paths: Personalized learning journeys that evolve with a person’s career and interests.
Conclusion
Data science is transforming education by making it smarter, more personalized, and more inclusive. From predicting student success to improving content and supporting teachers, the possibilities are endless. As edtech continues to grow, data will be the driving force behind more effective and empowering learning experiences.
Whether in the classroom, online, or somewhere in between, data science is helping education reach its full potential.